Using AI and ML in Assay Development or Improvement - Image Analysis, our Favourite!
We like these papers because image analysis is just so impactful in assay development. Here are two exciting takes on new assays: 3D cell culture viability and lipid droplet analysis in biofuel production.
Nondestructive, quantitative viability analysis of 3D tissue cultures using machine learning image segmentation1
Developing their Segmentation Algorithm to Assess the ViabilitY (SAAVY), Trettner et al. aim to automatically identify and analyze critical parameters for viability measurement in 3D tissue cultures.
SAAVY utilizes deep learning, specifically a fine-tuned Mask R-CNN model, to automatically identify and analyze critical features in spheroid and organoid structures indicative of cellular viability, such as the transparency of the spheroid and the overall morphology. The algorithm demonstrates noise tolerance, providing accurate assessments even in the presence of common defects in 3D cultures.
The study validates SAAVY using pancreatic ductal adenocarcinoma (PDAC) spheroids and compares its performance against industry standards and human experts. SAAVY achieves comparable results to human expert estimation, outperforming an industry-standard metabolic assay (CellTiter-Glo) in certain aspects. Furthermore, SAAVY exhibits longitudinal tracking capabilities, allowing non-destructive monitoring of 3D cultures over time.
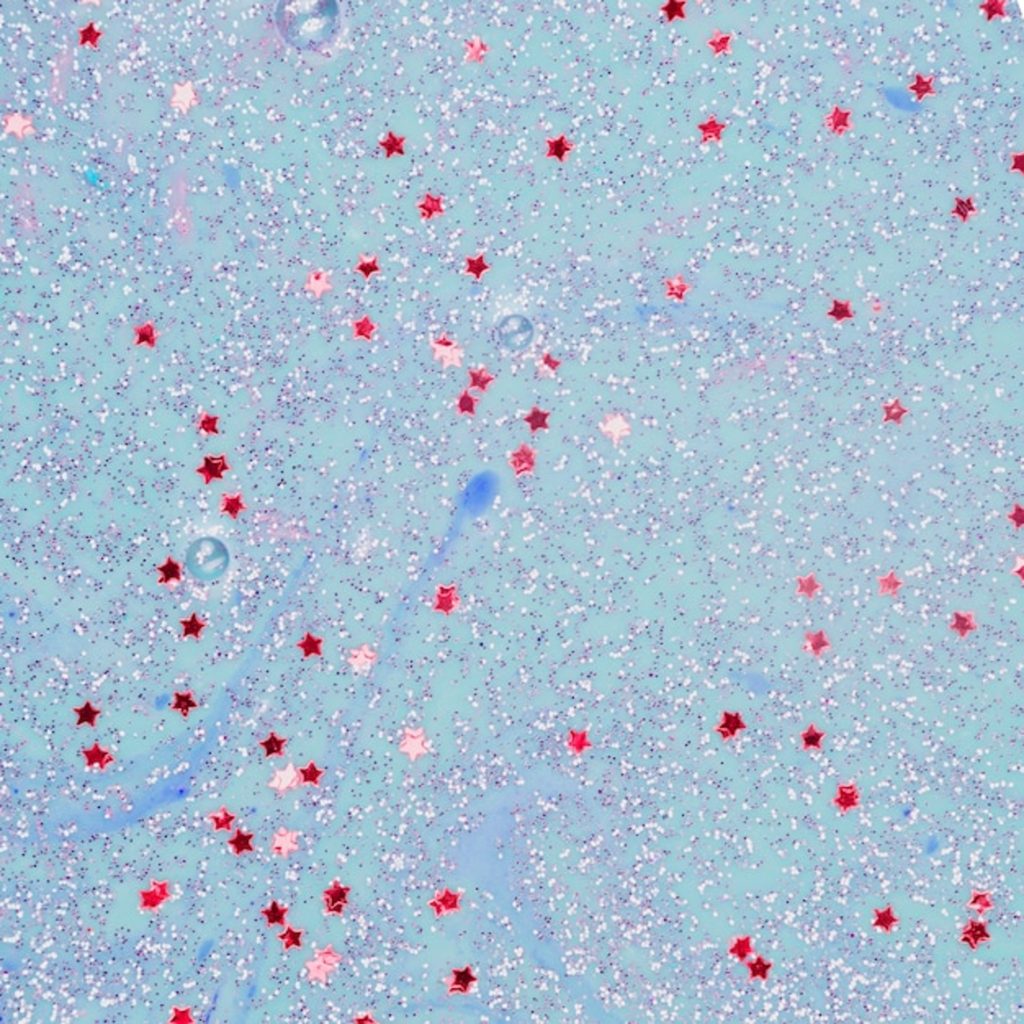
The paper concludes by highlighting the potential of SAAVY as a label-free, non-destructive, and longitudinal tool for viability assessments in 3D tissue cultures, offering valuable insights into growth dynamics and responses to therapeutic perturbations.
The algorithm’s versatility, agnosticism to microscope systems, and quick analysis time enhance its applicability in various experimental settings. Future directions include expanding the training dataset and exploring 3D imaging for a more comprehensive understanding of compound effects on overall growth dynamics.
Automated quantification of lipid contents of Lipomyces starkeyi using deep-learning-based image segmentation2
The study introduces a deep-learning-based segmentation model for the automated detection and quantification of intracellular lipid droplets (LDs) in the oleaginous yeast Lipomyces starkeyi, with significant implications for biofuel and dietary lipid production. LDs play a crucial role in long-term carbon storage and exhibit dynamic interactions with cellular compartments, making their real-time monitoring essential for biomass harvest and isolating promising strains.
The developed model achieved remarkable accuracy, detecting yeast cells and LDs in light microscopic images with 98% and 92% precision, respectively. This accuracy is especially notable given the challenges posed by the irregular features of cell and LDs in L. starkeyi. The model’s application extends to predicting lipid content, offering a streamlined and automated approach that significantly reduces cost and time in the real-time monitoring of lipid production.
The study’s emphasis on the use of deep learning for direct detection of visible LDs and quantification of lipid content distinguishes it from conventional brightfield image analysis, which often struggles with irregular morphologies. The approach addresses a gap in the field, where few studies have focused on direct detection of visible LDs and lipid content quantification. The model’s success is evident in its ability to predict lipid content in liquid cultures with high accuracy, comparable to results obtained through gas chromatography-mass spectrometry (GC–MS), a precise method for total lipid quantification.
In conclusion, the deep-learning-based segmentation model offers a promising advancement in the ex vivo dynamics and monitoring of LDs, presenting an efficient tool for bio-fermentation processes. The study showcases the potential of AI-driven image analysis in revolutionizing lipid production processes, with implications for biofuel and dietary lipid industries.
Using AI and ML for smart cell culture media and matrix generation - Wild!
We like these papers because it is really a novel idea to us to use ML for cell culture improvement, rather than more directly applied scientific predictions. Neat!
Challenges in developing cell culture media using machine learning3
This review explores the emerging role of machine learning (ML) in revolutionizing cell culture engineering by combining high-throughput experimental technologies to develop efficient culture media.
The paper emphasizes the importance of experimental design, data acquisition, model construction, medium prediction, and validation in the ML-assisted medium development process. The use of statistical methodologies such as design of experiments (DOE) for determining experimental conditions is recommended, ensuring a balance between abundance, variation, and experimental costs.
The review compares ML with conventional methodologies, which benefit from their simplicity, but have limitations in considering component interactions. ML, on the other hand, provides a more holistic approach, capable of handling large and complex datasets. The discussion underscores the need for practical searching strategies within computational constraints and time limitations when predicting novel medium compositions.
While ML has demonstrated superior performance in optimizing microbial cell cultures, its application to mammalian cell cultures remains an evolving area. The review discusses challenges specific to mammalian cell cultures, such as growth capacity, product yield, and cost bottlenecks. It emphasizes the potential of ML in addressing these challenges, particularly in the context of stem cell cultures for clinical and therapeutic purposes.
The review suggests that ML algorithms need further refinement and evaluation beyond the commonly used artificial neural networks (ANN). There is a need for upgrading ML algorithms to better suit medium optimization, considering the complexity of mammalian cell cultures.
Exploring the Potential of Artificial Intelligence for Hydrogel Development – A Short Review4
Irina Negut and Bogdan Bita’s review, “Exploring the Potential of AI for Hydrogel Development – A Short Review,” navigates the transformative influence of artificial intelligence (AI) and machine learning (ML) on hydrogel design. Focusing on applications in medicine and biotechnology, from drug delivery to tissue engineering, the authors advocate for the integration of AI into hydrogel research.
The review introduces the concept of “AI train hydrogel design,” decoding relationships between hydrogel compositions, structures, and properties. This approach integrates classical methods, setting the stage for advancements fueled by AI and ML.
AI has potential in accelerating material discovery, predicting hydrogel behaviour, and optimizing properties. It emphasizes interdisciplinary collaboration across materials science, chemistry, biology, and computer science.
AI incorporation revolutionizes hydrogel design, predicting properties, optimizing synthesis, and aiding regulatory compliance. ML, especially Random Forest, ANNs, SVMs, and DNNs, streamlines development, expediting formulation identification and property fine-tuning. Collaborative efforts between scientists and ML experts are crucial for advancing hydrogel design.
In conclusion, Negut and Bita’s review, alongside the synergistic integration of numerical, analytical, and AI-driven methods, encapsulates the multifaceted approach essential for propelling hydrogel research. The synthesis not only highlights the field’s current state but also emphasizes addressing challenges and ethical considerations to fully leverage AI’s potential in reshaping hydrogel applications in medicine and biotechnology.
Using AI and ML for Disease Prediction, Prognosis and Drug Development - Classic!
We like these papers because they show some recent advances in prognosis prediction and protein-protein interactions.
CSM-Potential2: A comprehensive deep learning platform for the analysis of protein interacting interfaces5
According to the authors of this paper, the CSM-Potential2 is a comprehensive deep learning platform that facilitates the study of protein interactions and their analysis in 3D structures. It is designed to provide easy access to state-of-the-art methods for both expert and non-expert users, ultimately enhancing our understanding of how proteins interact with various molecules. The platform is freely available as a web server and API.
It is important to understand protein structure and its relationship to biological function, particularly in light of recent advancements in protein structure prediction methods like RosettaFold and AlphaFold2. These advances have led to the development of various computational approaches to explore protein interactions. However, many of these methods are limited in their scope and complexity, making them less accessible to non-expert users.
The CSM-Potential2 is an updated platform that aims to address these limitations. It provides tools for the analysis of protein-protein interactions, prediction of interaction sites with nucleic acids, biological ligand classification, and ligand transplantation based on sequence and structure similarity to experimentally determined structures. The platform is available as a web server and API.
The paper highlights the four types of analysis offered by CSM-Potential2:
- Identification of protein-protein interaction (PPI) binding sites.
- Prediction of interaction sites with nucleic acids (DNA and RNA).
- Biological ligand classification, helping users identify the binding affinity of specific ligands to pocket regions on a given protein structure.
- Ligand transplantation using the AlphaFill algorithm, which allows users to transplant ligands from structurally similar proteins to a given protein structure.
The authors provide details about the data sources and training datasets used for developing the predictive models, including datasets derived from PPI databases, BioLiP, and others. The deep learning models used for PPI binding site prediction and nucleic acid binding site prediction are also described.
Diverse cell death patterns-related signature for predicting prognosis and drug sensitivity of osteosarcoma patients6
Programmed cell death (PCD) is a natural process in which cells undergo controlled self-destruction, which plays a crucial role in maintaining tissue homeostasis and eliminating damaged or unnecessary cells. The connection between PCD and osteosarcoma was explored in the present study.
Twelve types of PCD were collected for developing a prognostic signature in osteosarcoma using machine learning algorithms. The prognostic value, pathway annotation and drug prediction of the signature were explored.
Telomerase reverse transcriptase (TERT) was found to be a potent hazardous marker in osteosarcoma and could facilitate the proliferation and migration of osteosarcoma.
In summary, the present study has developed a prognostic signature for osteosarcoma and identifies TERT as a potent hazardous gene. The study suggests that further research is needed to address the underlying mechanism of how TERT affects the immune response in osteosarcoma.
[NOTE: Directly copied from abstract]
Leveraging a disulfidptosis-related signature to predict the prognosis and immunotherapy effectiveness of cutaneous melanoma based on machine learning7
In this study, the newly identified programmed cell death, disulfidptosis, exhibits promise in controlling cutaneous melanoma (CM) growth. Utilizing data from The Cancer Genome Atlas and other datasets, 18 key disulfidptosis-related genes (DRGs) were identified and analyzed for their genetic characteristics and transcriptional changes in CM. A protein-protein interaction network, somatic mutation evaluation, and DRG expression analysis in CM samples were conducted, revealing high DRG expression in CM tissues.
Two distinct disulfidptosis-related clusters with varying expression profiles and overall survival rates were identified. The predictive model was refined by screening 40 new DRGs, constructing a disulfidptosis-related signature for CM using various analysis methods. The resulting model significantly associated with patient survival, indicating a poorer prognosis in the high-risk group.
External validation across multiple datasets reinforced the robustness of the signature, consistently showing lower overall survival rates in the high-risk group. The signature also exhibited potential as a predictive tool for immunotherapy response, correlating with lower overall survival rates and increased risk scores.
Pathway and functional analyses highlighted biological functions associated with the signature, revealing enriched pathways in the high-risk group related to DNA replication and aminoacyl tRNA biosynthesis. The low-risk group showed activity in pathways such as antigen processing and presentation, cell adhesion molecules, and T cell receptor signalling.
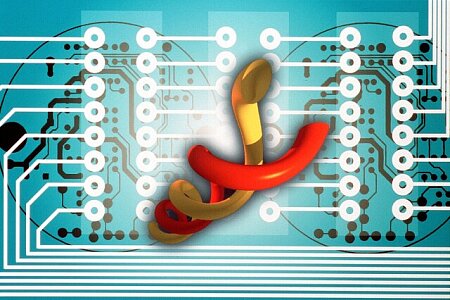
Reference List
- KJ Trettner et al.: Nondestructive, quantitative viability analysis of 3D tissue cultures using machine learning image segmentation. arXiv 2023, arXiv:2311.09354 [q-bio.QM]. doi: 10.48550/arXiv.2311.09354
- J-J Oh et al.: Automated quantification of lipid contents of Lipom.yces starkeyi using deep-learning-based image segmentation. Biores. Tech. 2024, 393, 130015. doi: 10.1016/j.biortech.2023.130015.
- T Hashizume and B-W Ying: Challenges in developing cell culture media using machine learning. Biotechnology Advances, 2024, 70, 108293. doi: https://doi.org/10.1016/j.biotechadv.2023.108293.
- I Negut and B Bita: Exploring the Potential of Artificial Intelligence for Hydrogel Development—A Short Review. Gels 2023, 9(11), 845. doi: 10.3390/gels9110845.
- CHM Rodrigues and DB Ascher: CSM-Potential2: A comprehensive deep learning platform for the analysis of protein interacting interfaces. Proteins, 2023, Oct 23. doi: 10.1002/prot.26615.
- Y Shi et al.: Diverse cell death patterns-related signature for predicting prognosis and drug sensitivity of osteosarcoma patients. J Gene Med. 2023, e3613. doi: 10.1002/jgm.3613.
- Y Zhao et al.: Leveraging a disulfidptosis-related signature to predict the prognosis and immunotherapy effectiveness of cutaneous melanoma based on machine learning. Mol. Med., 2023, 29, 145. doi: 10.1186/s10020-023-00739-x